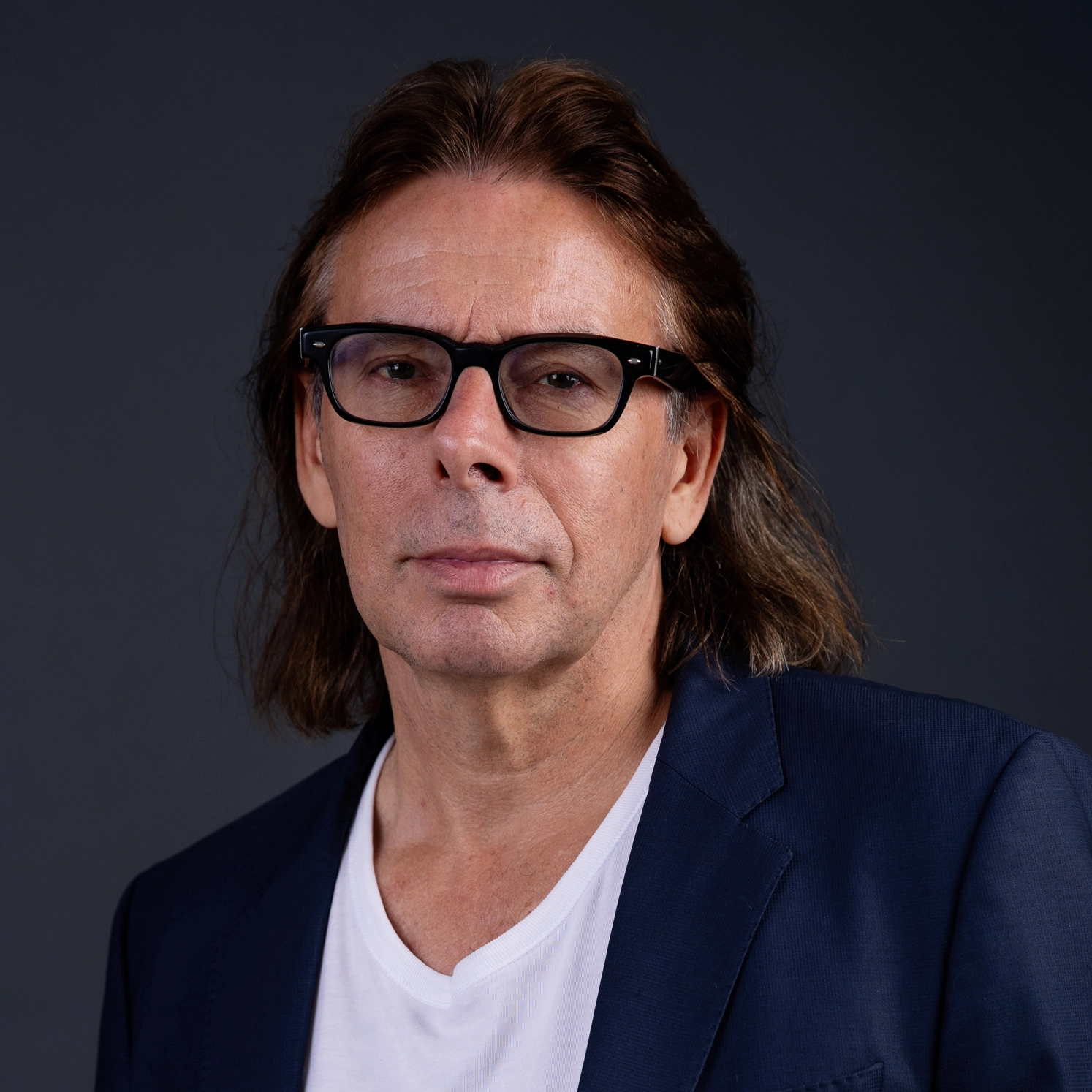
Live and Learn in Scenic Cambridge
Enrol Here
- –
- 5 dias (participação flexível)
- Churchill College, University of Cambridge
Join us this July in Cambridge for a 5-day in-person Econometrics Summer School designed to equip researchers, analysts, and policy professionals with cutting-edge tools for causal inference and machine learning using Stata.
Hosted at Churchill College, the summer school brings together a community of researchers, students, and professionals from around the globe and offers a rare opportunity to study cutting-edge methods in causal inference and machine learning in one of the world’s most prestigious academic centres.
Participants will not only benefit from expert-led instruction and hands-on Stata sessions, but also experience life in a Cambridge college—with optional on-site accommodation available. In the evenings, participants will have the opporunity to unwind with fellow attendees over a drink or explore the vibrant university town with its winding lanes, bookshops, and riverside walks. Stay tuned for more updates on evening social activties organised for all attendees.
The programme is made up of two complementary 2.5-day courses, led by internationally renowned instructors and structured to build progressively from foundational methods to more advanced machine learning applications. Participants may choose to register on the whole school or on each course independently. The two courses have been structured to flow from one to the other, providing a seamless learning experience.
In addition, all participants are invited to an evening panel discussion and drinks reception on Day 3, offering an opportunity to engage in informal discussion with instructors and peers in one of the world’s most iconic academic settings.
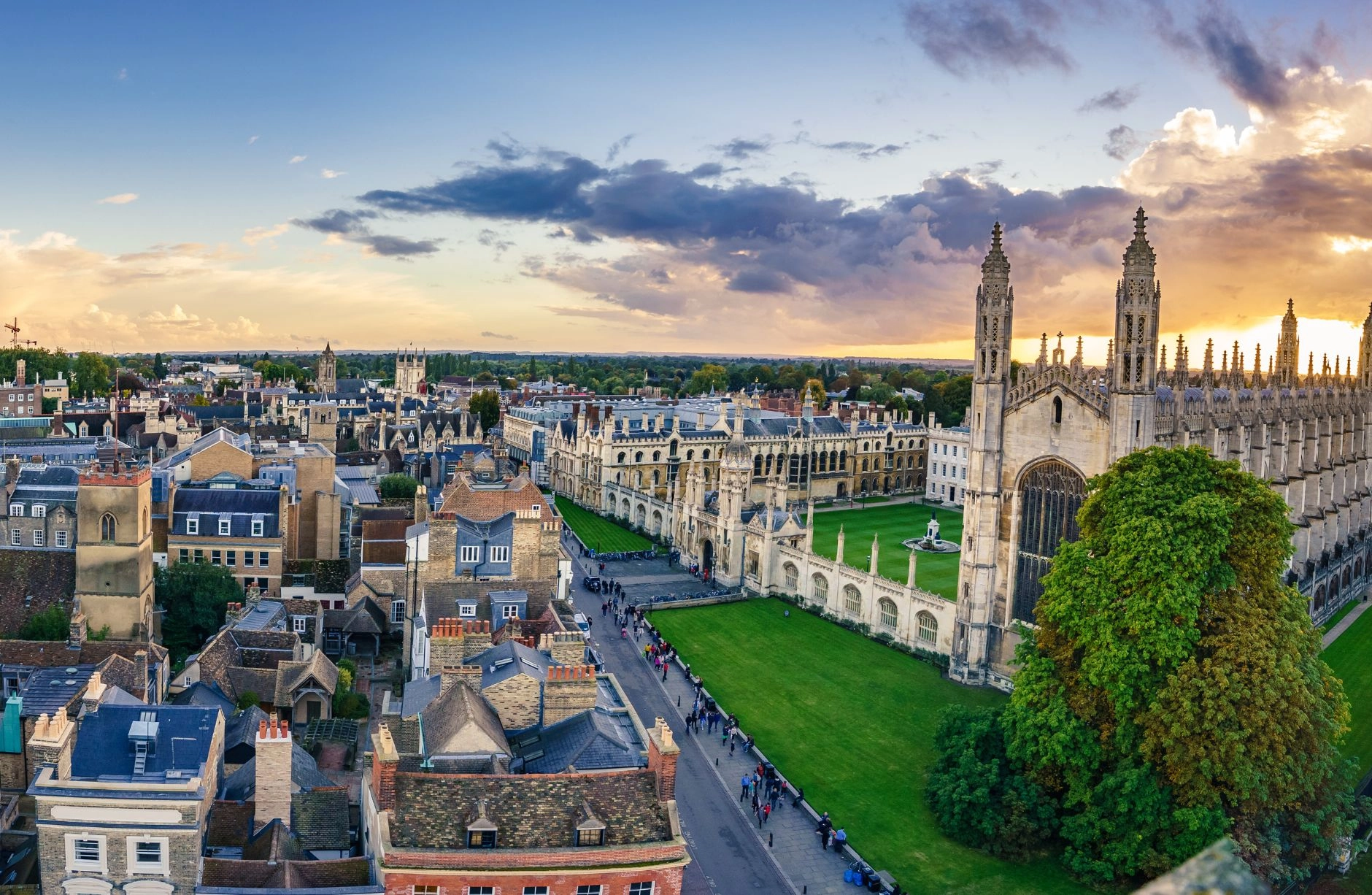
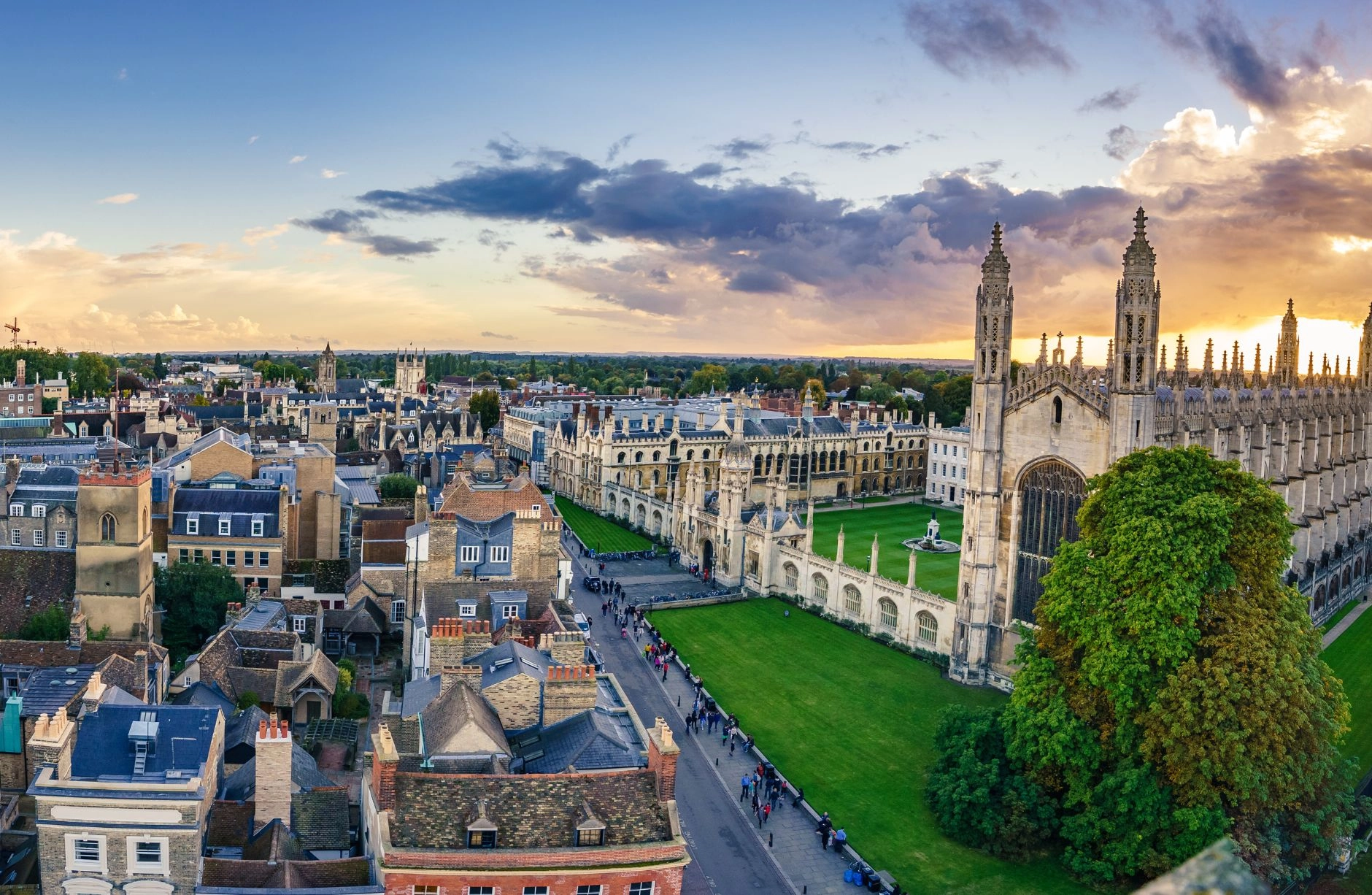
Course Structure
Course One: An Introduction to Causal Inference and Difference-in-Differences using Stata
InstructorProfessor Jeffrey Wooldridge (University of Michigan)
Duration2.5 Days
LevelIntermediate–Advanced
This course provides a rigorous introduction to causal inference using the potential outcomes framework. You’ll explore both traditional and modern estimation techniques for treatment effects in cross-sectional and panel data settings.
Topics Include:
-
Regression adjustment, inverse probability weighting, and matching
-
Doubly robust methods and covariate balancing techniques
-
Local average treatment effect (LATE) and LATT estimation
-
Control function methods with heterogeneous treatment effects
-
Difference-in-differences (DiD): leads/lags, staggered adoption, parallel trends testing
-
Advanced DiD topics: clustered standard errors, few groups, rolling methods
Course Two: Causal Inference and Machine Learning using Stata
InstructorDr. Melvyn Weeks (University of Cambridge)
DurationDays 2.5–5
LevelAdvanced
This course explores the intersection of machine learning and causal inference, helping participants move beyond prediction-focused applications to unlock the full potential of ML in policy and economics research.
Topics Include:
-
Contrasting econometrics and machine learning: Breiman’s “two cultures”
-
Ensemble methods: bagging, random forests, regularisation, averaging
-
ML for causal inference: double-robust methods, double-debiased Lasso, orthogonalisation
-
Frisch-Waugh-Lovell theorem & Lasso for treatment effect estimation
-
Parametric vs nonparametric methods in treatment effects
-
Managing high-dimensional data and sample splitting
New for 2025: Generative AI & Unstructured Data
-
Intro to LLMs (e.g., GPT-4, BERT)
-
Sentiment extraction from central bank speeches
-
Application to stock market response analysis
Evening Panel Discussion & Reception – Day 3
All attendees are invited to a panel discussion featuring both instructors, with additional guests from academia and industry. This will be followed by a drinks reception, offering a relaxed setting for networking and discussion.
What You'll Take Away
- Confidence using Stata for exploratory analysis, data visualisation, and statistical modelling.
- Practical experience through worked examples, take-home materials, and Q&A sessions.
- Skills that are transferable across disciplines – while examples are health-focused, the principles apply broadly.
Learning Outcomes
- Apply inverse probability weighting, matching, and doubly robust methods using Stata
- Implement advanced DiD designs, including staggered treatment and dynamic effects
- Diagnose and address violations of the parallel trend’s assumption
- Estimate and interpret LATE and LATT in observational studies
- Handle clustering and small-sample inference issues in applied work
Why Attend?
-
Learn directly from Jeffrey Wooldridge, one of the most cited econometricians in the world
-
Explore machine learning for causal inference with Melvyn Weeks, an expert on applied econometrics and ML
-
Gain hands-on experience with Stata
-
Develop skills applicable across academia, policy, and industry
-
Build your professional network in the unique atmosphere of Cambridge
Accomodation
Participants may choose whether to register for the school either inclusive or non-inclusive of accomodation. All accomodation is provided at Chuchill College, University of Cambridge and all rooms are double rooms allowing single occupancy.
Accomodation is included for the evenings between course days, for example, if you book accomodation for Course 1 you will recieve a room on the nights of the 21 & 22 July only. Similarly if accomodation is booked for Course 2, you will receive a room for the nights of the 23 & 24 July only. Those who book on the whole school will receive all 4 nights.
If you require accomodation for either Sunday 20 July or Friday 25 July, please contact us, we may also be able to arrange this onsite subject to availability and additional cost.
Social Schedule and Industry Panel
Get ready to balance learning with some brilliant social events! This year’s programme isn’t just about stats and study—we’ve lined up a series of fun, relaxed, and enriching experiences to help you connect and unwind.
Midway through the course, join us for an exclusive Panel Session and Reception, where you’ll hear from leading industry voices sharing how they use the techniques you’re learning in the real world.
Here's what’s in store:
- Monday 21 July 2025: Pub Quiz Night
Kick off the week with some friendly competition at our classic British pub quiz. Test your trivia skills and get to know your fellow participants. - Wednesday 23 July 2025: Panel Discussion & Drinks Reception
Hosted at the prestigious Churchill College, this evening brings together experts from industry and academia for an insightful discussion, followed by a relaxed reception—your chance to network and ask questions. - Thursday 24 July 2025: Punt Tour on the River Cam
Round off the week in true Cambridge style with a scenic punt tour along the River Cam. Glide past the city’s most iconic colleges and bridges as you soak up the summer sun and charming atmosphere.
Course 1: An Introduction to Causal Inference and Difference - in -Differences using Stata
Overview
Instructor: Prof. Jeffrey Wooldridge, Michigan State University
Date: 21 - 23 July 2025
Duration: 2.5 Days
Level: Intermediate - Advanced
This course covers causal inference for cross-section and panel data using the potential outcomes framework. We will cover identification of key treatment effects assuming unconfoundedness, and then study various estimators: regression adjustment, inverse probability weighting, combinations thereof (to achieve double robustness), and matching methods. More recent methods using covariate balancing to obtain the propensity score also will be covered. We will then study identification of the local average treatment effect (LATE) and the local average treatment effect on the treated (LATT) and cover recent doubly robust methods for estimating these parameters when one has covariates in the instrument propensity score. Control function methods can be used to allow heterogeneity when functional forms are imposed on the conditional mean functions.
We then turn to difference-in-differences methods for panel data, starting with the common timing case with possibly many periods before and after the intervention. We will focus initially on regression-based methods, studying both “lags only” and “leads and lags” models.
For the general staggered intervention case, we will discuss how to allow full heterogeneity in treatment effects by treatment cohort and calendar time. Various estimators turn out to be equivalent, including an extended version of two-way fixed effects. Covariates are easily incorporated. Testing and adjusting for violation of parallel trends in the staggered case will be covered. Rolling methods and long differencing, where one can apply standard treatment effects, will also be covered.
Additional special topics include allowing for exit from treatment, clustering standard errors, and inference when there are few control or treated groups.
Participants should have good working knowledge of ordinary least squares estimation, fixed effects estimation, and basic nonlinear models such as logit, probit, and exponential conditional means. Sufficient background is provided by my introductory econometrics book, Introductory Econometrics: A Modern Approach, 7e, Cengage, 2020. My book Econometric Analysis of Cross Section and Panel Data, 2e, MIT Press, 2010, covers the background material at a higher level.
Some of the material is based on my recent working papers, “Two-Way Fixed Effects, the Two-Way Mundlak Regression, and Difference-in-Differences Estimators,” and my Econometrics Journal paper, “Simple Approaches to Nonlinear Difference-in-Differences.” Some of the recent material on doubly robust and matching estimators is in the paper “A Simple Transformation Approach to Difference-in-Differences Estimation for Panel Data” (with Soo Jeong Lee).
Day 1 - 21 July 2025
Session 1: 9:00-10:30
Potential outcomes and parameters of interest. Randomization. Unconfoundedness and overlap. Identification. Regression adjustment.
Break: 10:30-10:45
Session 2: 10:45-12:15
Inverse probability weighting, normalized weights. Covariate balancing propensity score estimation. Matching and doubly robust estimators. Improved efficiency under randomized controlled trials.
Lunch: 12:15-13:30
Session 3: 13:30-15:00
Potential treatment status. Constant effect model. Compliers and defiers. One-sided non-compliance. Identification of LATE and LATT. Including covariates for LATE and LATT estimation.
Break: 15:00-15:15
Practical: 15:15-17:00
Q&A: 17:00-17:30
Day 2 - 22 July 2025
Session 4: 9:00-10:30
Control function methods. Regression discontinuity (time permitting).
Break: 10:30-10:45
Session 5: 10:45-12:15
Difference-in-Differences with Common Timing. Parameters, no anticipation, parallel trends.
Lunch: 12:15-13:30
Session 6: 13:30-15:00
Difference-in-Differences with Staggered Interventions. No anticipation, parallel trends. Imputation and flexible regression-based methods. All units eventually treated.
Break: 15:00-15:15
Practical: 15:15-17:00
Q&A: 17:00-17:30
Day 3 - 23 July 2025
Session 7: 9:00-10:30
Event study methods with staggered interventions. Heterogeneous trends. Testing no anticipation.
Break: 10:30-10:45
Session 8: 10:45-12:15
Small number of treated units. Synthetic control and synthetic difference-in-differences.
Course 2: Causal Inference and Machine Learning using Stata
Overview
Instructor: Dr. Melvyn Weeks, University of Cambridge
Date: 23 - 25 July 2025
Duration: 2.5 Days
Level: Advanced
The course will focus upon topics at the intersection of machine learning and econometrics, covering a mix of theory and applications. In making the distinction between models which are used to solve a prediction problem and models which are used to estimate some form of causal effect, we demonstrate how empirical strategies such as unconfoundedness, instrumental variables, and difference-in-difference can be used alongsidemachine learning methods for prediction.
Using Breiman’s (2001) notion of two cultures in the use of statistical modelling, the course begins with a review of the fundamental differences between machine learning and econometrics.
In this context we contrast a modelling approach where the analyst makes certain assumption on model specification, including functional form, with an approach where the data mechanism is presumed unknown. In this context we consider the econometrician’s concern for internal validity, alongside the focus within machine learning of ensuring that a model is robust in the sense of generalising to unseen data (external validity).
According to Splunk’s latest report, The Economic Impact of Data Innovation 2023, 67% of data innovation leaders strongly agree that their data is growing faster than they can keep up. However, there can also be a problem of “too much data”. ml models use big data to learn and improve predictability and performance automatically through experience and data. Participants will be introduced to the use of ensemble methods such as simple averaging and other regularization devices, that are able to manage the problems associated with having too much data.
In this context we will explore a number of general methods for model averaging including bootstrap sampling (so-called bagging) and random forests.
Day 3 - 23 July 2025
Session 1: 13:30-15:00
Introduction, Machine Learning: The Vernacular, Causal Inference in Low Dimensions
Break: 15:00-15:15
Session 2: 15:15-17:00
The Best Predictor and The Conditional Expectation Function, Linear Regression, Nonlinear in Variables, Departing From The Linear cef: Wages and Education
Day 4 - 24 July 2025
Session 3: 9:00-10:30
Prediction, Estimation and Attribution, Prediction Policy Problems, Beyond Prediction and Attribution: Causal Effects, Estimation and Inference for Causal Effects, An Introduction to Treatment Effect Model, Anticipating Heterogeneous Causal Effects, Weak Instruments in Nonparametric Settings, Binary Response and Endogeneity
Break: 10:30-10:45
Session 4: 10:45-12:15
High-Dimensional Methods for Linear Models, Least absolute Shrinkage and Selection
Lunch: 12:15-13:30
Session 5: 13:30-15:00
Applications of Regularised Regression for Linear Models
Break: 15:00-15:15
Session 6: 15:15-17:00
Double Machine Learning, The Partial Linear Model (plm), Regularisation Bias Revisited, Orthogonalisation and Sample Fitting, A Decomposition of Bias, Variations on the plm, Stata Practical: The Impact of Institutions on Growth
Q&A: 17:00-17:30
Day 5 - 25 July 2025
Session 7: 9:00-10:30
Treatment Effects: ate and cate, Identification Strategies, Estimators, Regression Adjustment, Inverse propensity score weighting, AIPW and Double Robust Estimators, Double Robust Estimators Meet Machine Learning
Break: 10:30-10:45
Session 8: 10:45-12:15
Random Forests, Machine Learning and Decision Trees, Machine Learning for Prediction
Lunch: 12:15-13:30
Session 9: 13:30-15:00
The Architecture of Causal Trees and Generalised Random Forests, Why not use Off-the Shelf Methods for Prediction? From Random to Causal Forests, Infeasible MSE, Sample Splitting, Honest Estimation, From Hard Partitioning to Forest-Based Weights, Generalised Random Forests
Break: 15:00-15:15
Session 10: 15:15-17:00
Testing for Heterogenous Treatment Effects
Q&A: 17:00-17:30
Time permitting there will also be an Introduction to Generative AI and Large Language Models
Course Timetable
Terms
- Student registrations: Attendees must provide proof of full time student status at the time of booking to qualify for student registration rate (valid student ID card or authorised letter of enrolment).
- Additional discounts are available for multiple registrations.
- Delegates are provided with temporary licences for the principal software package(s) used in the delivery of the course. It is essential that these temporary training licenses are installed on your computers prior to the start of the course.
- Payment of course fees required prior to the course start date.
Cancellations
- 100% fee returned for cancellations made over 28-calendar days prior to start of the course.
- 50% fee returned for cancellations made 14-calendar days prior to the start of the course.
- No fee returned for cancellations made less than 14-calendar days prior to the start of the course.
The number of attendees is restricted. Please register early to guarantee your place.
Thinking of joining us in Cambridge this summer?
Get a head start with a free on-demand pre-course webinar hosted by Jeffrey Wooldridge.
We’re offering a complimentary online session ahead of the Econometrics Summer School in Cambridge to introduce key themes that will be explored in depth during the programme.
Date/Time: On-Demand
Format: Online via Zoom
Speaker: Professor Jeffrey Wooldridge
What to Expect:
This two-hour webinar provides a concise review of the method of Ordinary Least Squares (OLS) for cross-sectional data—still the foundational tool in modern econometrics, even in more complex causal inference settings.
Professor Wooldridge will present a contemporary perspective on OLS, asking a critical question: What can we learn when our model is only an approximation? He will cover:
-
Motivation for OLS and its Real-World Applicability
-
Conditional Expectations and Linear Projections
-
Statistical Properties of OLS
-
Practical Challenges in Applying OLS
Additional discussion will include functional form issues, interpreting partial effects when they vary, and addressing common sources of confusion such as multicollinearity.
Register below to access the session recording!
Validate your login
Sign In
Create New Account