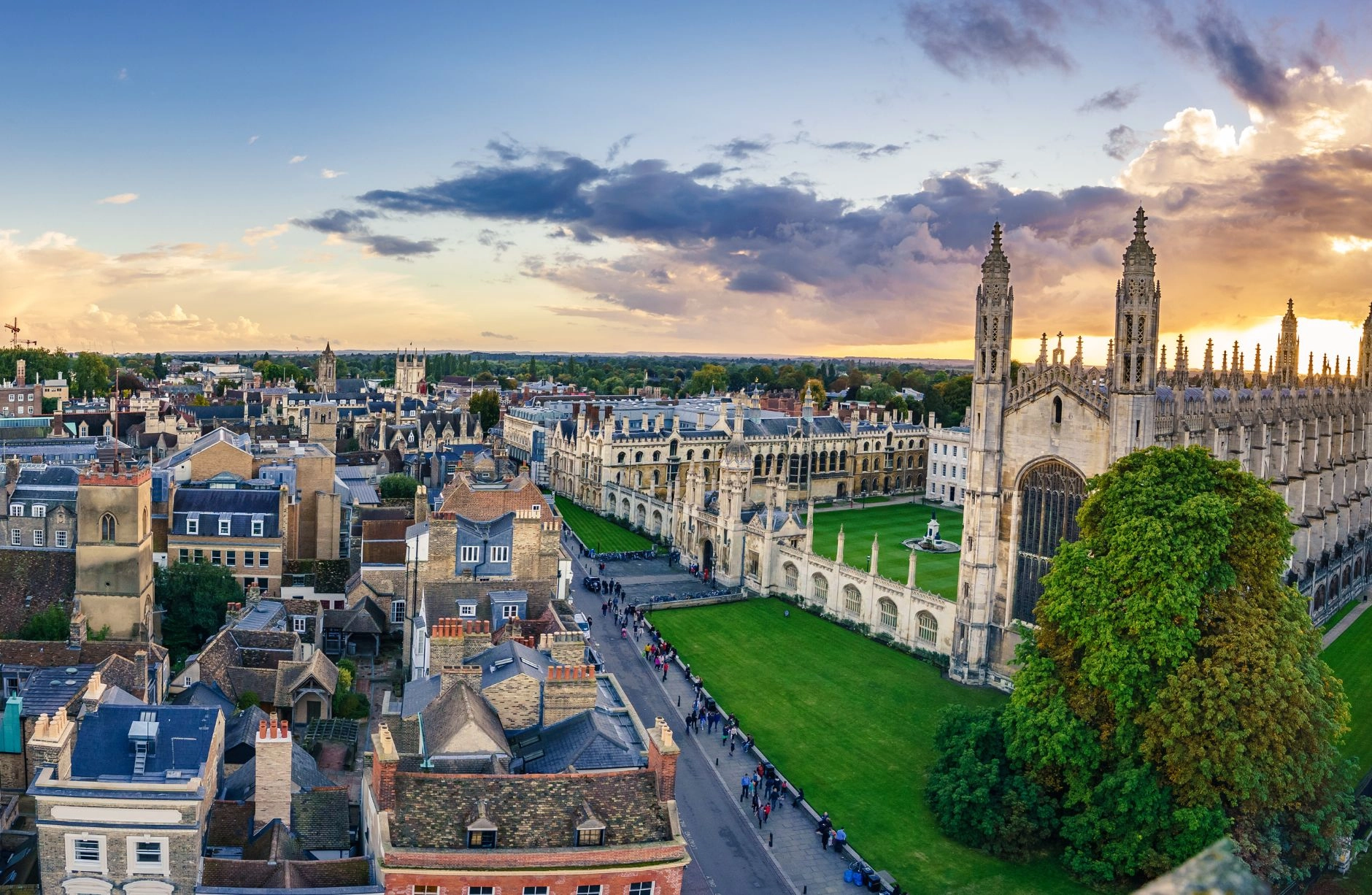
Data Science: A Necessity, Not an Option
Data science is no longer an option but a necessity
In this evolving landscape, data science is no longer an option but a necessity. By leveraging insights from real-time analytics, predictive models, and interdisciplinary data integration, data science supports both economic stability and health equity, making it a powerful tool in public health’s modernisation journey.
Public health presents a unique opportunity to drive meaningful impact
For professionals skilled in data and technology, public health presents a unique opportunity to drive meaningful impact. The integration of platforms like Stata and Python allows for robust, real-time analysis that enables public health experts to make evidence-based decisions and proactively address public health challenges in a constantly changing environment
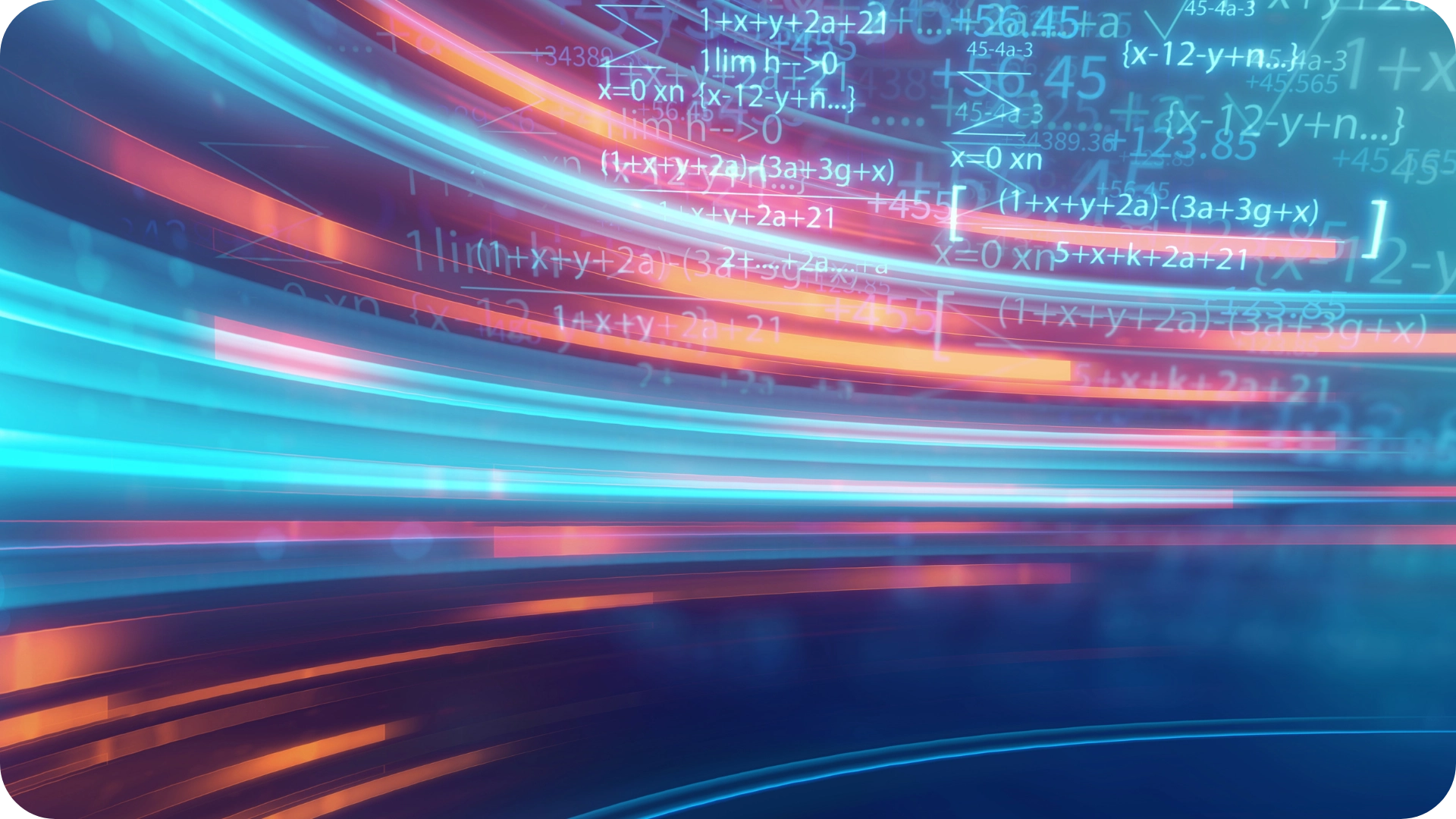
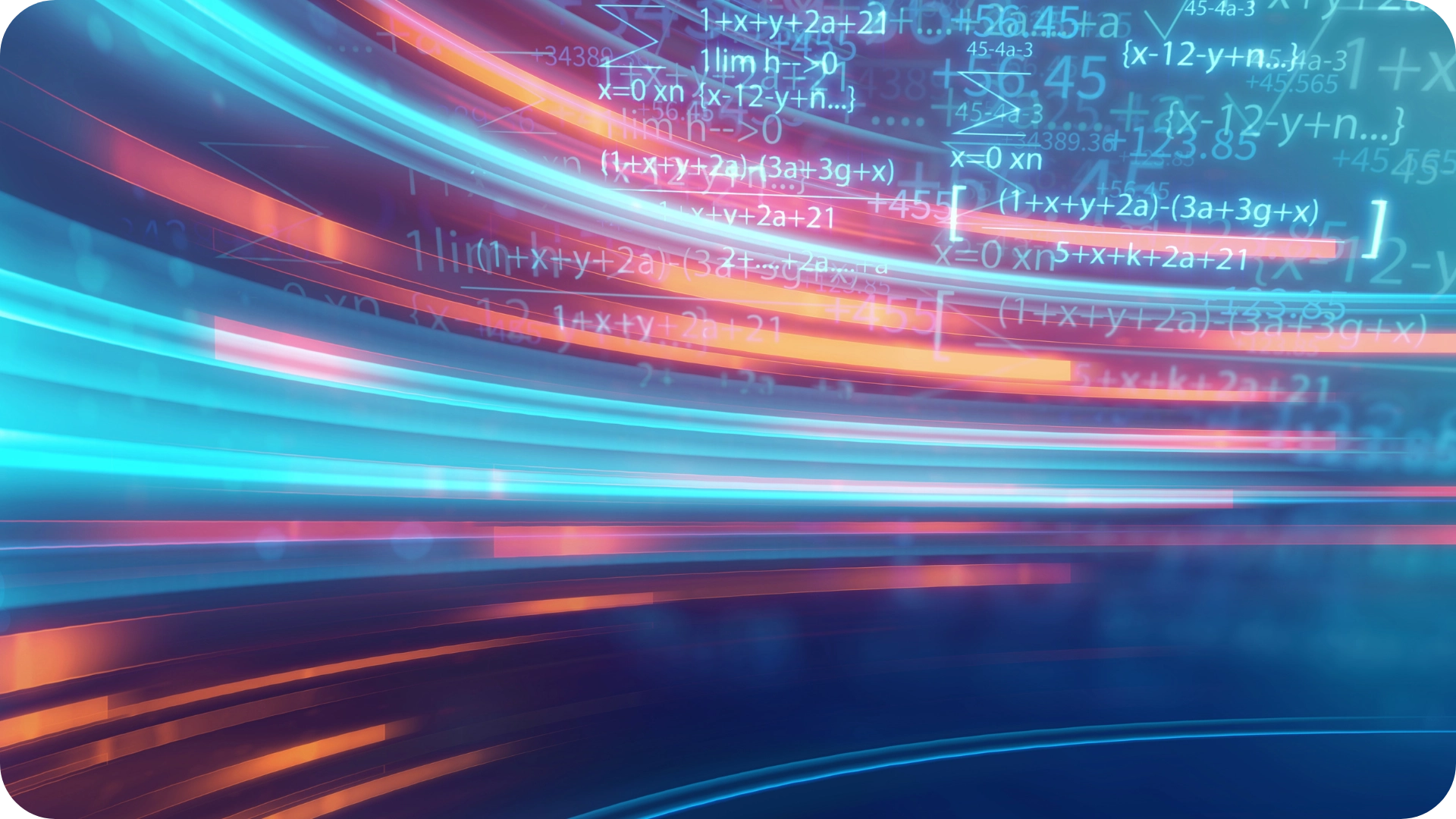
Harnessing Data Science for Real-Time Public Health and Economic Recovery Analysis with Stata and Python Integration
Understanding Data Science
“Did you know data-driven health insights have reduced outbreak response times by over 40%?”.
Data science is an interdisciplinary field that combines techniques from machine learning, artificial intelligence (AI), statistics, and high-performance computing to extract meaningful insights from complex datasets.
It enables public health professionals to collect, integrate, and analyse data from numerous sources, leading to actionable insights for better decision-making and intervention design (Mooney, 2018).
In the context of public health, data science facilitates early detection of infectious diseases, predictive modelling for outbreaks, and optimized resource allocation during health crises.
For example, advanced machine learning models can process vast amounts of real-time data, identifying health threats earlier and with greater precision than traditional methods. AI algorithms are also invaluable in analysing data from social media, health records, and environmental factors to identify emerging health trends and pre-emptively inform intervention strategies.
The Data Revolution in Precision Public Health
Technological advancements now enable the collection and integration of data from an expanding range of sources, such as genomic sequencing, satellite imaging, electronic health records, GPS-enabled devices, personal sensors, and smartphone applications (Prosperi, 2018).
This integration offers a holistic view of health dynamics, allowing for a deeper understanding of how biological, environmental, and lifestyle factors interact to influence public health.
The reduced cost of computing power and the increased capacity for data storage have made processing and analysing these large datasets more accessible than ever (Pearson, 2020). Precision public health harnesses this data-driven approach to design targeted interventions tailored to the specific needs of communities.
By integrating diverse data, public health professionals can monitor population health trends, pinpoint emerging risks, and develop data-driven solutions that address the root causes of health disparities (Khoury, 2016). The powerful combination of Stata and Python further enhances this capability, providing a seamless pipeline from data collection to statistical analysis.
Integrating Stata and Python: A Powerful Public Health and Economic Toolset
Integrating Stata and Python creates a powerful toolkit for real-time public health and economic analysis. Python’s extensive libraries excel at automated data collection, real-time web scraping, and predictive modelling, making it ideal for gathering and preparing data. Analysts can use Python to track daily COVID-19 cases, vaccination rates, or economic indicators, readying this data for further study.
Stata complements Python by providing robust statistical and econometric tools for detailed analysis, such as time-series modelling to examine connections between health metrics (e.g., infection rates) and economic indicators (e.g., GDP, unemployment). Its strengths include managing structured data, applying advanced models, and adjusting for seasonality and trends, essential for accurate policy insights.
Together, Python’s data processing and Stata’s statistical rigor enable analysts to build models that support both immediate public health responses and informed long-term policies.
Applications in Public Health and Economic Recovery
Integrating Stata and Python empowers critical public health and economic analysis, producing real-time insights that help design targeted interventions.
By simulating the economic impacts of health policies—such as vaccination campaigns, lockdowns, and social distancing—on indicators like GDP, unemployment, and consumer spending, decision-makers can better navigate the trade-offs involved in protecting public health while maintaining economic stability.
Python’s ability to gather, process, and automate real-time data, combined with Stata’s econometric modelling capabilities, provides a dynamic toolkit for simulating intervention outcomes, allowing policymakers to make empirically driven adjustments as conditions evolve.
Decision-makers can identify the strategies that yield the best results
This integrated approach also supports real-time evaluation of interventions aimed at mitigating health and economic risks. Governments and NGOs, for instance, can use these tools to assess the effectiveness of financial interventions like healthcare funding, social support programs, or subsidies. By tracking how these resources influence both economic resilience and public health outcomes, decision-makers can identify the strategies that yield the best results, enabling timely adjustments based on solid data.
Stata and Python's integration is equally powerful in public health surveillance. Predictive models can analyse disease transmission rates, monitor pathogen behaviour, and anticipate outbreaks, allowing for rapid intervention.
Machine learning algorithms in Python can analyse data from social media, news sources, and health records to detect emerging health issues, while Stata’s robust econometric tools can evaluate the long-term economic and health impacts of these early interventions.
Together, this constructive collaboration of tools enables a responsive, data-informed approach to addressing today’s complex public health and economic challenges.
The Future of Digital Public Health: Research Priorities
To advance digital transformation in public health meaningfully, research must go beyond theoretical discussions and guide the practical planning, deployment, and evaluation of digital health initiatives. A pressing question remains: "How can digital health tools be effectively scaled in resource-limited settings?".
Despite growing support for digital health, real-world applications in public health remain underdeveloped, particularly in low- and middle-income countries, where digital tools could have a transformative impact (Odone, 2019). Targeted research can help bridge this gap by identifying scalable models that address local health challenges and are adaptable across diverse healthcare systems.
One crucial area for future research is the economic modelling of health interventions. By analysing how policies—such as lockdowns, vaccination campaigns, or social distancing measures—affect broader economic indicators like employment rates, GDP, and consumer spending, researchers can provide policymakers with a more comprehensive understanding of intervention trade-offs.
Thus, Python’s data integration capabilities, paired with Stata’s statistical and econometric modelling tools, enable researchers to simulate policy impacts on the economy, refining forecasts and improving policymakers' ability to make data-informed decisions.
Future research should also investigate the effectiveness of digital tools and predictive analytics on health outcomes. Studies in this area could examine which digital health strategies yield the highest impact, providing evidence on how data-driven methods influence health resource allocation and outcomes. By identifying the most effective strategies, researchers can help shape a digital health roadmap that prioritises solutions that can be scaled effectively, particularly in settings with limited infrastructure.
Additionally, precision public health represents an emerging area of research focused on targeted interventions based on population-level data. By harnessing big data to predict health risks, researchers can help refine methods for identifying high-risk groups and determining which interventions can provide the most benefit. This approach, supported by Stata and Python's integration, can enhance models for detecting health disparities, identifying at-risk populations, and assessing intervention outcomes in real-time. The automation capabilities of Python, combined with Stata’s robust statistical analysis, allow for continuous refinement in public health practices, helping professionals adapt to evolving health and economic challenges effectively.
By pursuing these priorities, digital public health research can provide valuable insights and actionable data that are crucial for building resilient health systems, especially in the face of future global health crises.
Conclusion
The integration of Stata and Python forms a powerful toolkit for advancing public health and economic recovery through data science.
Together, these tools streamline real-time data collection, advanced statistical analysis, and predictive modelling, which are all crucial for creating resilient, data-informed systems.
By equipping professionals to handle data with efficiency and precision, this combination supports the development of policies and interventions that are responsive to current needs and adaptable for future challenges.
Mastering Stata and Python empowers data scientists and public health professionals to make a tangible impact on critical decisions in public health, economic stability, and community resilience.
This expertise enables informed, proactive responses to emerging crises and promotes an agile approach to resource allocation, health monitoring, and economic recovery.
As we navigate an era of complex global challenges, the ability to leverage real-time, data-driven insights is not only valuable but essential for building healthier, more resilient societies.
So, what role do you envision data science playing in the future of public health?
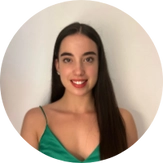
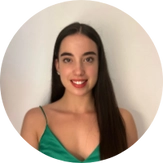
Francisca Carvalho, Lancaster University
Francisca is a third-year PhD student in Economics at Lancaster University. Her research focuses on climate risk factors and their impact on portfolio returns. She also teaches mathematics, econometrics, macroeconomics and microeconomics, to undergraduate and postgraduate students.
- Khoury, M. J. (2016). Precision public health for the era of precision medicine. American journal of preventive medicine, 50(3), 398.
- Mooney, S. J. (2018). Big data in public health: terminology, machine learning, and privacy. Annual review of public health, 39(1), 95-112.
- Odone, A. B.-M. (2019). Public health digitalization in Europe: EUPHA vision, action and role in digital public health. European journal of public health, 29(Supplement_3), 28-35.
- Pearson, T. A. (2020). Precision health analytics with predictive analytics and implementation research: JACC state-of-the-art review. Journal of the American Co.
- Prosperi, M. M. ( 2018). Big data hurdles in precision medicine and precision public health. BMC medical informatics and decision making, 18, 1-15.
Related Posts
-
Cambridge 2025
-
Stata 19 training
Why Timberlake is the ideal Partner to deliver Structured Hands-on Training with Stata 19
-
AI: The Future of Labour Markets
-
Farewell GPT-4.0: Which is the best ChatGPT Model for Econometrics & Data Science Programming?
-
The Green Economy: Can Sustainable Investments Drive Growth?
-
The Interest Rate Rollercoaster: How High Will They Go?
Privacy Overview
This website uses cookies to improve your experience while you navigate through the website. Out of these cookies, the cookies that are categorized as necessary are stored on your browser as they are essential for the working of basic functionalities of the website. We also use third-party cookies that help us analyze and understand how you use this website. These cookies will be stored in your browser only with your consent. You also have the option to opt-out of these cookies. But opting out of some of these cookies may have an effect on your browsing experience.
Essential
Name | Description | Lifetime |
---|---|---|
ADD_TO_CART | (Adobe Commerce only) Used by Google Tag Manager | 1 Year |
GUEST-VIEW | Stores the Order ID that guest shoppers use to retrieve their order status. Guest orders view. Used in Orders and Returns widgets | 1 Year |
LOGIN_REDIRECT | Preserves the destination page that was loading before the customer was directed to log in | 1 Year |
MAGE-BANNERS-CACHE-STORAGE | (Adobe Commerce only) Stores banner content locally to improve performance | 1 Year |
MAGE-MESSAGES | Tracks error messages and other notifications that are shown to the user | 1 Year |
MAGE-TRANSLATION-STORAGE | Stores translated content when requested by the shopper | 1 Year |
MAGE-TRANSLATION-FILE-VERSION | Tracks the version of translations in local storage | 1 Year |
PRODUCT_DATA_STORAGE | Stores configuration for product data related to Recently Viewed/Compared Products | 1 Year |
RECENTLY_COMPARED_PRODUCT | Stores product IDs of recently compared products | 1 Year |
RECENTLY_COMPARED_PRODUCT_PREVIOUS | Stores product IDs of previously compared products for easy navigation | 1 Year |
RECENTLY_VIEWED_PRODUCT | Stores product IDs of recently viewed products for easy navigation | 1 Year |
RECENTLY_VIEWED_PRODUCT_PREVIOUS | Stores product IDs of recently previously viewed products for easy navigation | 1 Year |
REMOVE_FROM_CART | (Adobe Commerce only) Used by Google Tag Manager | 1 Year |
STF | Records the time messages are sent by the SendFriend | 1 Year |
X-MAGENTO-VARY | Configuration setting that improves performance when using Varnish static content caching | 1 Year |
FORM_KEY | A security measure that appends a random string to all form submissions to protect the data from Cross-Site Request Forgery | 1 Year |
MAGE-CACHE-SESSID | The value of this cookie triggers the cleanup of local cache storage | 1 Year |
MAGE-CACHE-STORAGE | Local storage of visitor-specific content that enables ecommerce functions | 1 Year |
MAGE-CACHE-STORAGE-SECTION-INVALIDATION | Forces local storage of specific content sections that should be invalidated | 1 Year |
PERSISTENT_SHOPPING_CART | Stores the key (ID) of persistent cart to make it possible to restore the cart for an anonymous shopper | 1 Year |
PRIVATE_CONTENT_VERSION | Appends a random, unique number and time to pages with customer content to prevent them from being cached on the server | 1 Year |
SECTION_DATA_IDS | Stores customer-specific information related to shopper-initiated actions, such as wish list display and checkout information | 1 Year |
STORE | Tracks the specific store view/locale selected by the shopper | 1 Year |
Marketing
Name | Description | Lifetime |
---|---|---|
CUSTOMER_SEGMENT_IDS | Stores your Customer Segment ID | 1 Year |
EXTERNAL_NO_CACHE | A flag that, indicates whether caching is on or off | 1 Year |
FRONTEND | Your session ID on the server | 1 Year |
GUEST-VIEW | Allows guests to edit their orders | 1 Year |
LAST_CATEGORY | The last category you visited | 1 Year |
LAST_PRODUCT | The last product you looked at | 1 Year |
NEWMESSAGE | Indicates whether a new message has been received | 1 Year |
NO_CACHE | Indicates whether it is allowed to use cache | 1 Year |
Functionality
Name | Description | Lifetime |
---|---|---|
MG_DNT | Allows you to restrict Adobe Commerce data collection if you have custom code to manage cookie consent on your site | 1 Year |
USER_ALLOWED_SAVE_COOKIE | Used for cookie restriction mode | 1 Year |
AUTHENTICATION_FLAG | Indicates if a shopper has signed in or signed out | 1 Year |
DATASERVICES_CUSTOMER_ID | Indicates if a shopper has signed in or signed out | 1 Year |
DATASERVICES_CUSTOMER_GROUP | Indicates a customer's group. This cookie is stored as sha1 checksum of the customer's group ID | 1 Year |
DATASERVICES_CART_ID | Identifies a shopper's cart actions | 1 Year |
DATASERVICES_PRODUCT_CONTEXT | Identifies a shopper's product interactions. This cookie contains the customer's unique quote ID in the system | 1 Year |
Statistical
Name | Description | Lifetime |
---|---|---|
_ga | Used by Google Analytics | 1 Year |
_ga_* | Used by Google Analytics | 1 Year |
Validate your login
Sign In
Create New Account